Two frameworks for understanding the risks and opportunities of AI-driven power line inspections
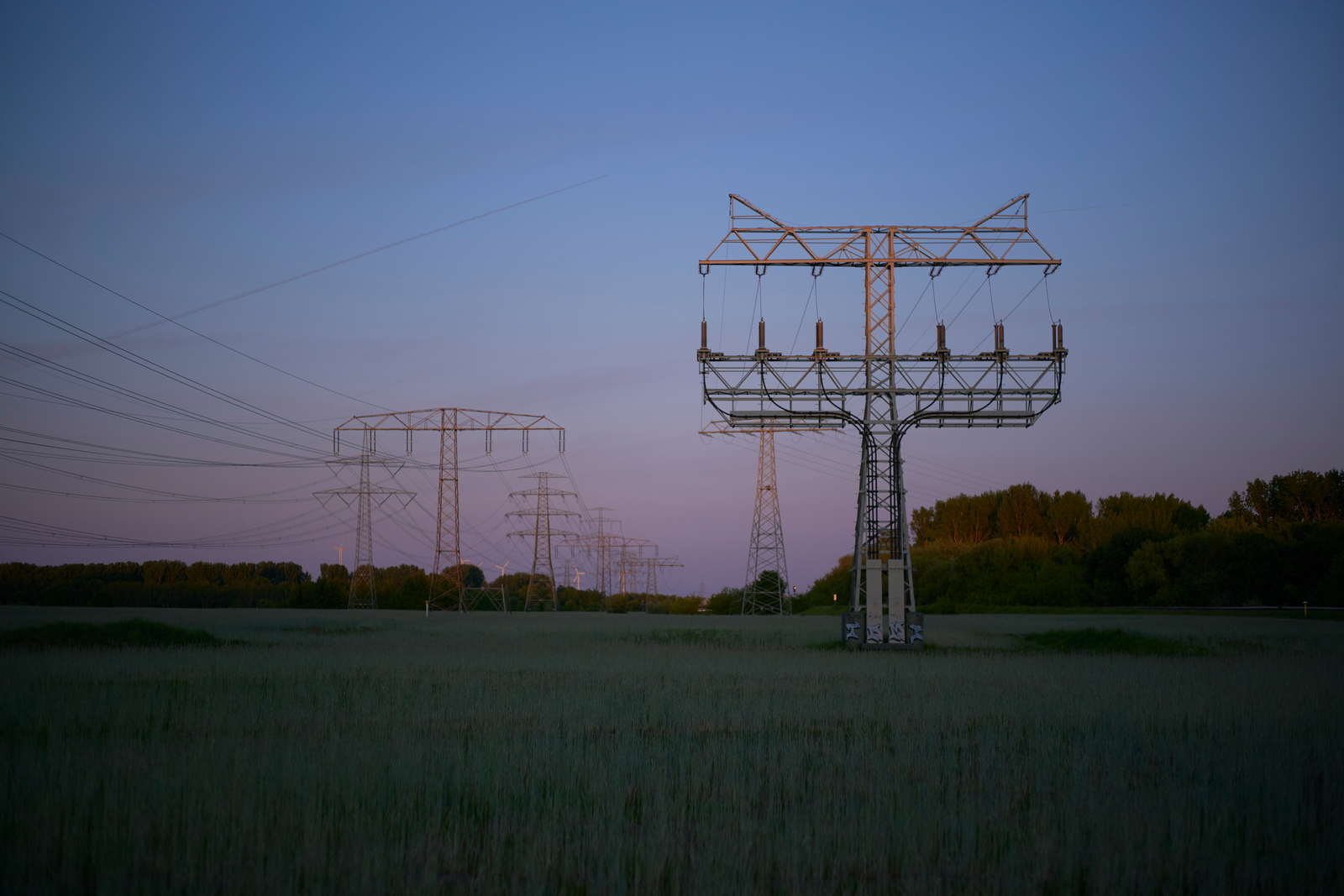
Background
The power grid is one of the most vital infrastructures in modern society, yet failures in grid assets result in annual costs of $150 billion—around 0.15% of global GDP. This number continues to rise as demand on the grid grows. Despite this, traditional inspection methods—such as manual checks, helicopters, and ground crews—remain slow, costly, and highly susceptible to human error. In fact, these methods fail to detect up to 90% of critical defects.
With climate challenges, aging infrastructure, and growing regulatory demands, grid operators can no longer afford to rely on reactive maintenance. A shift toward preventive strategies, powered by AI-driven insights, is essential for ensuring resilience, efficiency, and long-term grid stability.
The way forward is clear—drone inspections combined with AI-powered image analysis. This method provides the fastest, most cost-effective, sustainable, and, above all, precise way to monitor, assess, and maintain transmission and distribution grids.
Many transmission and distribution operators are beginning to embrace AI-driven maintenance with great success. E.ON, for example, has been leveraging this approach for years, while others have adopted it for specific use cases, such as post-storm assessments. However, the industry as a whole remains slow to adapt.
While the benefits of AI are many, many operators hesitate due to concerns about technology readiness. Others dive in without a clear strategy, leading to fragmented, small-scale implementations that struggle to scale—ultimately hindering the full potential of AI.
This cautious approach to change could become a major challenge for power grids over the next 20 years, as demand is expected to double, renewables dominate the energy mix, and the grid rapidly ages. A new approach is essential. As Faisal Hoque from Harvard Business Review puts it:
"Bridging the gap between aspiration and achievement requires a systematic approach to AI transformation, one that primes organizations to think through the biggest questions this technology raises without losing sight of its day-to-day impact. The stakes could not be higher. Organizations that fail to adapt will become the Polaroids and Blockbusters of the AI age."
The Frameworks
The world cannot afford for power grids to become the Polaroids or Blockbusters of the AI age; they must continue delivering reliable, affordable, and sustainable energy. To bridge this gap, a structured, balanced approach to AI adoption is necessary. Two complementary frameworks can help guide grid operators: the OPEN and CARE frameworks.
The OPEN framework (Outline, Partner, Experiment, Navigate) equips grid operators with a systematic four-step process to integrate AI into their workflows, enabling a smoother transition from proofs of concept to large-scale implementation.
The CARE framework (Catastrophize, Assess, Regulate, Exit) provides a ‘sanity check’ to map and manage AI-related risks while aligning adoption with existing organizational guardrails.
To achieve meaningful progress toward scaled AI deployments across the grid, energy stakeholders must first ask: What is preventing adoption, given that the technology is already live, tested, and delivering value to industry peers?
AI adoption is not just a technology or innovation project—it requires cross-departmental alignment and effort. A dual mindset of acceleration and risk mitigation is key to unlocking the efficiency, cost, and precision gains that AI can deliver.
Applying the Frameworks in Real Life
In this article, these frameworks are applied to critically assess how AI-powered asset analytics for power line inspections fit within these models.
The New Method for Power Line Inspections
Traditional power line inspection methods—manual checks, helicopters, and ground patrols—are slow, costly, and often fail to detect defects. AI-powered asset analytics fundamentally transform this process by integrating advanced technologies to enhance efficiency, accuracy, and decision-making.This is the process Arkion follows.
- Data Collection– Drones or helicopters capture high-resolution images, thermal images and 3D LiDAR data of the power grid assets and lines.
- AI and Computer Vision Analysis – Machine learning models analyze images and 3D data to detect defects such as corrosion, missing components, and vegetation encroachment. AI can process thousands of images in minutes, identifying patterns that would take human inspectors days to review.
- Human Verification – AI insights are augmented and cleaned by expert validation. Human inspectors review flagged anomalies, ensuring that critical decisions are based on both automated insights and experienced judgment.
- Actionable Insights and Integration – The analyzed data integrates into asset management and work order systems, enabling predictive maintenance, risk mitigation, and optimized investment planning.
Benefits of AI-powered Inspections
- Cost-Effectiveness: Using case studies from the industry, an average cost reduction per defect found is calculated to 85%.
- Precision: AI-driven analysis detects defects at a rate of up-to 5 times more compared to manual inspections, and on many more problematic or hard to inspect stretches, up-to 8 times more defects are found.
Despite these advantages, resistance persists among key stakeholders, from maintenance and vegetation teams to executives. This is where structured frameworks like OPEN and CARE help align different departments within grid owner organizations to accelerate AI adoption.
Applying the OPEN Framework to AI-Driven Power Line Inspections
The OPEN framework emphasizes that successful adoption depends not only on technology but also on leadership and a culture capable of sustaining continuous transformation. Each step in the process enables organizations to manage AI projects from ideation to deployment, maintenance, and eventual scaling from project to fully implemented.
Step 1: Outline
Define how AI adoption increases grid reliability and resilience.
A common mistake in AI adoption is treating it as a tech-first initiative. Instead, grid owners should ask: How does AI-driven inspection align with the value we deliver to society? The question is not "What can this technology do for us?" but rather "What can this technology do to help us deliver safe, reliable energy?"
How AI aligns with core grid operations:
- Maintenance Teams: AI rapidly analyzes drone-captured images to detect corrosion, missing bolts, damaged insulators, and overheating components before failures occur.
- Vegetation Management Teams: AI-powered analytics identify encroaching vegetation, enabling proactive trimming and reducing fire risks.
- Asset Management & Investment Teams: AI-driven condition assessments inform long-term infrastructure planning, preventing costly reactive maintenance.
- IT & Security Teams: AI models must integrate securely with existing grid management systems, ensuring compliance and data protection.
Internal priorities should be mapped and openly discussed so AI adoption is viewed through the lens of ensuring a resilient, steady energy supply.
Step 2: Partner
Build Cross-Functional and External Collaborations.
When AI is reframed from a tech initiative to a business value initiative, internal and external collaboration becomes essential. The most common pitfall is reviewing the tech and not the dependencies and process needed to fully utilize the technology. To avoid the constant evaluation of partners, some clear parameters and goals need to be set on both internal resources and goals, as well as the external partners that can help with technical expertise and change management.
- Look to your peers: Transmission and distribution power grid operators have undertaken similar projects at various scales. Learning from these examples is crucial, as they offer valuable insights into both opportunities and potential pitfalls. Engage with potential external partners—ask who they are working with and request discussions with those involved. One of the greatest strengths of the power grid industry is its collaborative nature. Sharing best practices—of which there are many—can accelerate positive change across markets.
- Internal Collaboration: Grid operations, IT, vegetation, and maintenance teams all benefit from using the same data and approach, creating strong synergies. However, achieving alignment doesn’t mean combining all goals at once. Keep the long-term vision in mind when identifying partners, but maintain a focused scope to ensure measurable results and clear evaluations.
- Vetting External Partners: AI providers should be assessed based on reference cases, domain expertise, security compliance, and their ability to scale with the grid owner’s needs. This often requires looking beyond local markets or conventional tech vendors. A successful partner must combine deep industry knowledge with technical expertise. Consider both transmission and distribution sectors, identify overlapping challenges, and seek reference cases that address multiple verticals.
- Establishing Governance: Clearly define business ownership, compliance requirements (e.g., GDPR), and whether AI capabilities should be developed in-house or outsourced. Given the critical nature of power grid infrastructure, governance is essential. Implement relevant ISO certifications, rigorous security reviews, and structured oversight processes with regular check-ins and "toll gates" to ensure quality and mitigate risk.
- Human-AI Synergy: AI should be positioned as an enabler, enhancing human expertise rather than replacing it. In power line inspections, the goal is to maximize the time internal teams spend addressing actual issues rather than sifting through raw data. AI should streamline workflows, prioritize actions, and accelerate resolutions.
By fostering strong internal and external collaboration, grid owners can accelerate AI adoption and scale its benefits efficiently.
Step 3: Experiment
Pilot AI-driven inspections in controlled environments.
Grid owners hesitant about AI adoption often fall into two camps: those who delay due to uncertainty and those who launch small-scale tests without clear objectives. Both approaches can hinder progress. Instead, organizations should adopt a structured experimentation approach that balances quick wins with long-term scalability.
Key considerations for experimentation:
- Start with a focused use case. Identify a high-impact, measurable problem—such as detecting defects on insulators or identifying vegetation encroachment.
- Define clear success criteria. Establish key performance indicators (KPIs) like defect detection accuracy, cost savings, and inspection speed improvements. These should be evaluated not only against your goals but also against the baseline of what is currently being detected. While technical success is often measured against a 100% accuracy rate, real business value comes from practical impact. Pursuing perfection can sometimes mean overlooking meaningful improvements. This report explores how AI model evaluations for power grids can be structured effectively.
- Run pilot programs. Test AI performance in real-world conditions where baseline data—such as the number of issues found in manual inspections—is well understood.
- Measure, iterate, and scale. Use pilot results to refine AI models, workflows, and integration processes before full deployment. Continuously expand by adding new use cases and refining evaluation criteria. However, in power grid operations, scale is the ultimate challenge. While early iterations should be nimble, long-term success depends on the ability to scale solutions effectively. The real test lies not just in delivering accuracy on a small scale but in ensuring AI can operate efficiently across vast networks.
Organizations that take a structured, hypothesis-driven approach to AI experimentation avoid costly missteps while ensuring that pilots lead to tangible, scalable benefits.
Step 4: Navigate
Scale AI Adoption with a Long-Term Roadmap
The final step in AI adoption is moving from pilots to full-scale implementation, which requires a clear roadmap aligned with business goals, regulatory requirements, and workforce readiness. This also involves managing large datasets to deploy AI at scale. If the previous steps have been done correctly, the main challenge here will be scaling the process, not the AI development itself. A well-defined roadmap ensures seamless integration into operations, addressing both short-term needs and long-term objectives.
Key Steps for Navigating AI Adoption
- Align AI with Grid Modernization Strategies
AI should be part of a broader digital transformation plan, not a standalone project. This integration ensures AI complements infrastructure upgrades and supports smarter grid management, maximizing synergies across technologies and departments. - Invest in Training and Change Management
Effective AI adoption requires training field staff, maintenance teams, and vegetation departments on how to interpret and act on AI-generated insights. Continuous learning and a culture of adaptability ensure employees are ready to embrace AI tools, improving safety, efficiency, and decision-making. - Continuously Improve and Adapt
AI models improve with more data. Establish feedback loops to regularly refine AI’s accuracy, efficiency, and reliability. This iterative process ensures AI-powered inspections evolve to detect issues early, optimize maintenance, and enhance grid performance.
Summary
The OPEN framework guides Transmission and Distribution operators through AI adoption by balancing technology, leadership, and organizational transformation. The best way to approach this is to take references, listen to your industry peers and ask how they handled these issues.
- Outline: AI adoption should align with core grid operations, improving reliability and resilience. Successful deployment involves all key teams—maintenance, vegetation management, asset planning, and IT—to ensure AI-driven inspections support long-term grid health.
- Partner: Cross-functional collaboration is critical. Internal teams must align on shared data usage, while external AI providers should be vetted for domain expertise, scalability, and security compliance. Clear governance structures help mitigate risks and ensure seamless integration.
- Experiment: Pilot programs should focus on high-impact use cases, such as defect detection and vegetation monitoring. Success is measured by improvements in accuracy, efficiency, and cost reduction. Iterative testing ensures AI scales effectively across large networks.
- Navigate: Scaling AI requires a structured roadmap integrated with grid modernization strategies. Workforce training, continuous model refinement, and feedback loops are essential for long-term success.
By following these steps, grid operators can scale AI adoption, future-proof their infrastructure, and fully unlock the benefits of AI-powered inspections. This approach ensures grids are more resilient, efficient, and ready for future challenges.
Applying the CARE Framework: Managing AI Risks in Power Line Inspections
While AI adoption offers transformative benefits, it also introduces risks that grid operators must proactively manage. The CARE framework provides a structured approach to addressing potential pitfalls.
Once again, a first and clear tip is to ask your peers. Review case studies, talk with other companies that have undertaken this sort of project and ask them the questions you should ask themselves. Their worries, and their approach to mitigate those risks, should form as a foundation to your approach as well.
Catastrophize: Identify Worst-Case Scenarios
Before deploying AI at scale, operators must ask: What is the worst that could happen? Potential risks include:
- False negatives leading to undetected critical defects.
- AI model bias causing inconsistent defect classification.
- Cybersecurity vulnerabilities exposing grid infrastructure data.
By anticipating worst-case scenarios, organizations can build safeguards into their AI strategy. These should also be compared with the baseline, what are the current % of missed critical defects? Is this worse or better than the alternative? Remember that business value is derived from real–life outcomes, so comparing with a technically perfect solution can very easily lead to decision paralysis. So ask the same question of your current process as of the new process, how do they compare? The answer lies in the gap between risks of your current inspection process and the hypothetical new process.
Assess: Evaluate Risk Impact and Probability
Not all risks carry the same weight. Grid operators should categorize AI risks based on likelihood and severity, prioritizing mitigation efforts accordingly.
Key risk areas to assess:
- Data quality: AI models depend on high-quality drone imagery and metadata.
- Model accuracy: Periodic validation against human inspections ensures reliability.
- Operational impact: Assess how AI-driven insights translate into actionable maintenance decisions.
Regulate: Implement Governance and Controls
AI adoption must align with industry regulations, cybersecurity protocols, and ethical guidelines. Grid operators should establish governance structures, including:
- AI model transparency: Clear documentation on how AI detects and classifies defects.
- Human oversight: AI insights should complement, not replace, human expertise.
- Compliance with data protection laws: Ensure AI systems meet GDPR, NERC CIP, and other relevant regulations.
Exit: Define Fallback Strategies
AI-driven inspections should not become a single point of failure. Organizations must have exit strategies in case AI models underperform, including:
- Maintaining manual inspection capabilities as a backup.
- Keeping flexibility in vendor partnerships to switch providers if needed.
- Implementing continuous AI model retraining to prevent performance degradation.
In the case of inspections, this is usually easily done by keeping a similar structure to inspect for the future. Having a previously existing process and adapting a new technology to that helps make sure you don’t lose talent or competence to fallback on if a solution can’t solve the problem or is too risky to pursue.
Conclusion: The Path Forward for AI in Grid Inspections
AI-powered power line inspections are no longer a futuristic concept—they are an operational necessity. Grid operators that successfully integrate AI into their workflows will achieve greater reliability, cost efficiency, and sustainability. However, achieving this transformation requires a dual approach: embracing innovation while proactively managing risks.
By applying the OPEN framework, grid operators can methodically scale AI adoption, ensuring alignment with business goals and operational workflows. Simultaneously, the CARE framework ensures that potential risks are identified, assessed, and mitigated before they become critical issues.
With a structured, balanced approach, AI can help the power grid evolve to meet the demands of the next century—ensuring safe, reliable energy delivery in an increasingly complex world.
Source: This article was based on the concepts and frameworks presented by Faisal Hoque, published in the online version of the Harvard Business Review, march 6, 2025 here: https://hbr.org/2025/03/two-frameworks-for-balancing-ai-innovation-and-risk